How artificial intelligence is making our world a better place
Google, Facebook and others fill their pockets with billions using our data. But artificial intelligence can do much more – for example ending world hunger.
During my studies in artificial intelligence I didn’t learn that much about how powerful computers are. Most of all, I learned that many things humans do are less complex than we think they are.
But what would the results look like if instead we asked:
What marketing and saving the world have in common
Collecting data, finding patterns and making predictions. These are the 3 steps that allow Google, Facebook and Co. to detect
Step 1: Collect data
Data is
Raw data alone does not lead to interesting insights – that’s what the next step is about.
Step 2: Detect patterns
The term
Compared to humans they have a crucial advantage: We usually detect patterns by means of two factors and a couple of observations. When we observe Italians passionately discussing spaghetti and risotto during our summer holiday we are fast to conclude: »Italians like to talk about food.« When, despite pouring rain during our weekend trip to Rotterdam, we encounter a handful of cyclists, we deduce: »Dutch people cycle a lot.« The result of these everyday statistics is inevitably an oversimplified view of reality.
In contrast, an artificial intelligence can evaluate data sets with millions of observations, taking into account numerous factors. Logically, in doing so, it is able to detect patterns that humans could never recognize. The last step reveals why this can be very useful.
Step 3: Make predictions
Once patterns are detected, the artificial intelligence can answer precise questions. For example: Which users like Katy Perry? Who are potential customers for a new gay bar? Who can be convinced by the educational program of the green party? The program will spit out a prediction, such as: »Middle-aged men that have many Facebook friends and play World of Warcraft are interested in Katy Perry.«
Such statements can be mysteriously precise and yet they are grounded on mere statistics, not fortune telling. Likewise, our political stance and sexual orientation is not left to chance: What we are interested in, has an effect on which links we follow. Which videos we watch on Youtube can be predicted by the data track we leave behind.
»Artificial Intelligence is no magic. It’s math!« – Jim Stolze, Founder of AI For Good
Tech companies such as Facebook like this, as precise predictions about human behavior are how they earn their money. Their customers can choose precisely which audience they want to target with their ads. Facebook receives 97% of its income through advertisement. For Alphabet, the mother company of
But just as our behaviour is predictable, other aspects of the world are predictable as well: An artificial intelligence can be fed other kinds of data about climate, capital distribution or streams of refugees, just as it is fed the data of your clicks online. Also in these data sets, it
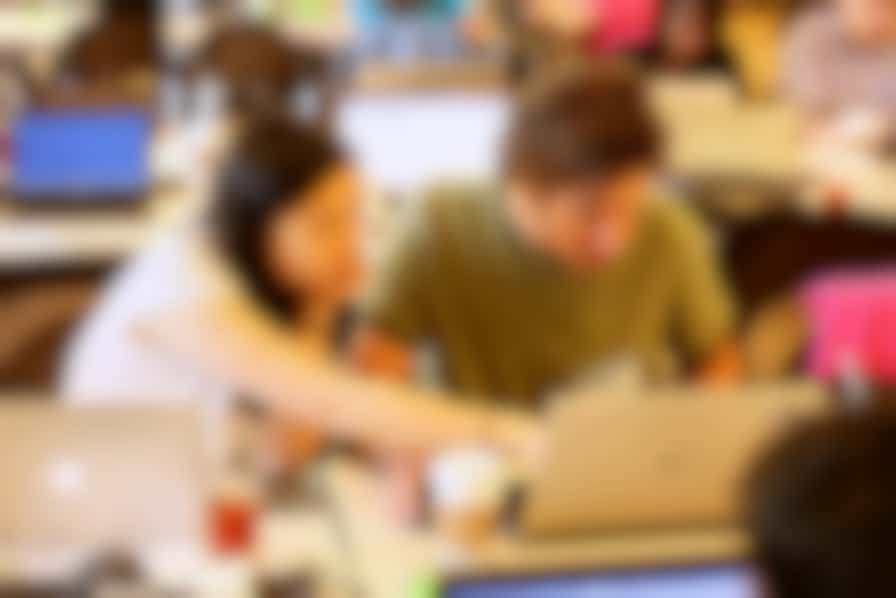
Data analysis meets activism
Jake Porway is the founder of the US-American start-up
Normally, many of the voluntary participants of the project work for renowned tech companies in which they deal with the typical applications of Big Data. In other words: advertisement. Their incentive to contribute to DataKind is the chance to use their abilities for a good cause instead of only generating clicks and profits.
Certainly, most problems cannot be solved on a weekend. However, the meetings are often the beginning of a larger process and encourage a rethink inside the NGOs. The organizations learn that they can take advantage of their data to improve their work.
In Europe, there are a growing number of companies and initiatives combining social activism and data analysis. Among these are the following 3:
- Model DataKind: In Berlin, the mathematician Daniel Kirsch founded the organization Data Science for Social Good
One of our biggest successes was the cooperation with the German Cancer Society. After working with us, they hired a data scientist to take care of their data. This is exactly the kind of change we want to bring about.
- Students solve problems: In Amsterdam, entrepreneur Jim Stolze founded the platform
- The UN faces the big issues: The program
We see data as a natural resource. It is available almost everywhere and always renewable.
All of this sounds very promising, but how does it work in practice?
Artificial intelligence for sustainable agriculture
Passionate environmental activist Tom shows us how the dream of a data driven activism can come true. In charity projects around the world he fights for
1. Stop deforestation
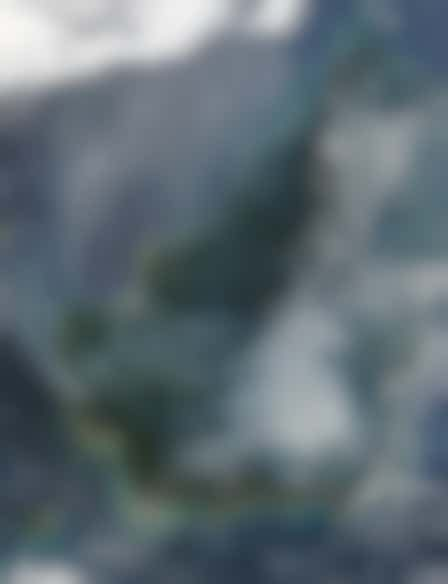
Tom:
Collect data: Andrea und Tom collect satellite data from the last decades. Using this data, they find out in which areas palm-oil plantations were created in the last years.
Detect patterns: Andrea combines the data about palm-oil plantations with information by the Indonesian government that depict which forest areas are protected. She designs a program that learns how the surroundings change before an illegal plantation is build, for example new roads might emerge through the jungle.
Make predictions: Now the program can predict where plantations are likely to be built in the future. On this basis, Tom’s organization can give precise advice to the Indonesian government: Which laws have to be adapted to prevent illegal deforestation and to protect the climate?
Who makes it possible? The platform
2. Less pesticides
Tom: »Many farmers use pesticides excessively without
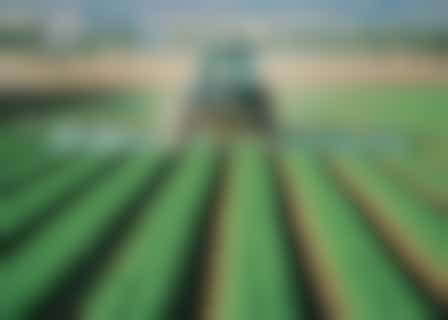
Collect data: Tom collects data from farmers throughout the world about the composition of their fields, soil conditions and crop diseases.
Detect patterns: Next, Andrea implements a program that detects patterns in these data. Which diseases are likely to develop under which conditions?
Make predictions: By means of this program, Andrea designs a tailored plan for every single farmer containing suggestions about how much of which pesticides and fertilizers he should use to optimize his harvest. With this tool, Andrea and Tom can achieve 2 effects: First, the farmers will harm the environment less, through reduced use of excessive or improper chemicals.
Who makes it possible? The founders of the Swiss company
3. Reduce food waste
Tom:
Collect data: Tom convinces one branch of a supermarket to participate in an experiment. He promises to reduce the amount of food wasted daily. In return, he obtains the supermarket’s sales statistics of the last 3 years.
Detect patterns: Using that data, Andrea designs a program that learns which products are bought every day. She doesn’t only input information about past sales, but also about other factors such as weather, season and offers from competitors into the program.
Make predictions: The program generates weekly predictions on the consumption of every single product the supermarket has on offer. The store manager starts to adjust his purchases to these forecasts. Soon, he recognizes the advantage of this procedure and other branches start adopting the same strategy to plan more efficiently. The program continues to learn and keeps improving its predictions. Thus over time, less and less products are wasted.
Who makes it possible? The start-up
»The interaction between man and machine is an important component of our work. We emphasize communication with employees instead of just providing them with a computer system that takes over their work.« – Tobias Pfaff, co-founder of FoodTracks
From field to store shelf –
Does that imply that machines should make decisions for us? By no means! The potential lies in combining human ideas and values on one side, and the precision of data on the other.
Titelbild: pixabay - CC0 1.0